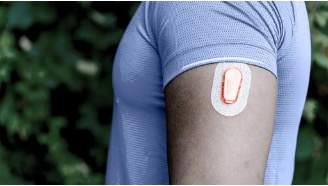
Development and implementation of integrated artificial intelligence models for Type 2 Diabetes risk prediction.
LINEA DE ACTUACIÓN 2: IMPLEMENTACIÓN Y ANÁLISIS DE BASES DE DATOS EN MEDICINA DE PRECISION.
Palabras clave: XXX
Coordina:
Participa:
Persona de contacto:
A large part of the health care burden in developed countries is devoted to the treatment and follow-up of patients with complex diseases, such as Type 2 Diabetes (T2D). For this reason, the identification of treatments, but above all of protocols for their prediction and prevention, have become priorities for research in biomedicine and for their translation into clinical practice. These diseases have a complex etiology, with a significant genetic burden and a strong dependence on external, environmental and lifestyle variables, the exposome.
A large part of the health care burden in developed countries is devoted to the treatment and follow-up of patients with complex diseases, such as Type 2 Diabetes (T2D). For this reason, the identification of treatments, but above all of protocols for their prediction and prevention, have become priorities for research in biomedicine and for their translation into clinical practice. These diseases have a complex etiology, with a significant genetic burden and a strong dependence on external, environmental and lifestyle variables, the exposome.
A large part of the health care burden in developed countries is devoted to the treatment and follow-up of patients with complex diseases, such as Type 2 Diabetes (T2D). For this reason, the identification of treatments, but above all of protocols for their prediction and prevention, have become priorities for research in biomedicine and for their translation into clinical practice. These diseases have a complex etiology, with a significant genetic burden and a strong dependence on external, environmental and lifestyle variables, the exposome.
Numerous genetic studies have identified hundreds of variations in the genome associated with the risk of developing this type of disease, with the promise and hope that their results would allow the identification of pharmacological targets, and the possibility of predicting the risk of suffering the disease. However, although they have targeted specific genes, the translation of this genetic information into predictive models with clinical validity is currently very limited. This is due to the poor predictive power of reductionist approaches, such as Polygenic Risk Scores, and the limited possibilities of integrating other clinical, environmental and lifestyle variables, which are potential risk modifiers in this type of pathology.
For this reason, in this project we propose the construction of prediction models for T2D, based on more integrative approaches using artificial intelligence (machine learning models), for the identification of genetic, clinical (variables and associated pathologies) and environmental determinants, and for the construction of prediction and classification models for T2D patients. With these combined models, we can not only propose new therapeutic targets, but also robust predictors that allow the development and implementation of lifestyle-based clinical prevention protocols for each individual.
COORDINA:
PARTICIPA:
COLABORA:
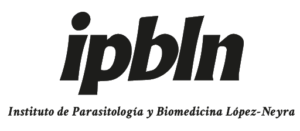
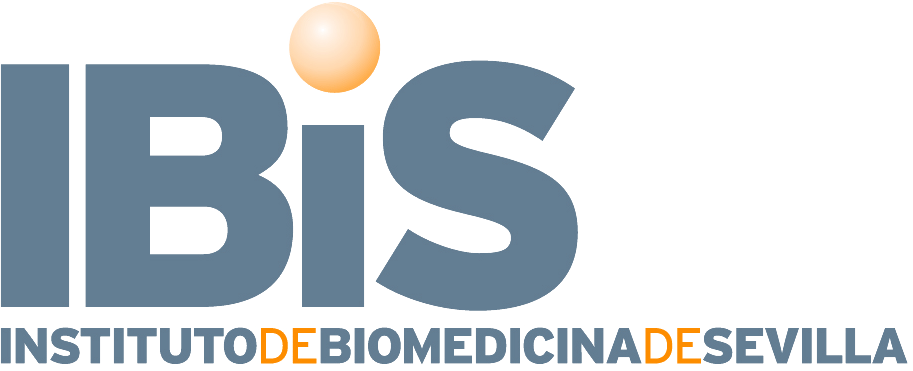